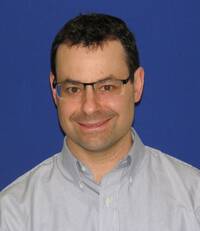
11 Dec Morbidity and Mortality Prediction Model Improved by Incorporating Data from Electronic Records
MedicalResearch.com Interview with:
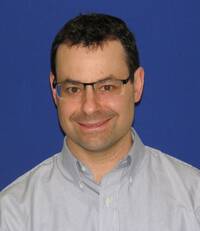
Prof. Adam Rose
Credt: Hebrew University
Prof. Adam J. Rose
Shuli Brammli-Greenberg and Adam J. Rose share senior authorship.
Faculty of Medicine, Hebrew University of Jerusalem
Beit-Horon, Jerusalem, 9093500, Israel
MedicalResearch.com: What is the background for this study? Would you briefly explain what is meant by the Elixhauser Comorbidity Model?
Response: Patients admitted to the hospital can have very different levels of illness severity. In addition, different hospitals may admit different numbers of very sick patients. Therefore, comparing two hospitals regarding something like length of stay or in-hospital mortality is not valid unless one adjusts for the illness burden of the population of patients at each hospital.
Risk adjustment is the name for the process of building a model to predict the risk of each patient for a particular outcome, such as mortality or readmission, based on what is known about them and their illness burden. By summing all the risks of patients at a hospital, one gets an aggregate sense of the illness burden at the hospital, and different hospitals can be compared.
The Elixhauser Comorbidity Model is a widely-used risk adjustment model which performs well in the sense that it is very predictive of outcomes like mortality. It also has the advantage of being calculated from diagnosis codes, which are widely available data for hospitalized patients.
MedicalResearch.com: What did your team do?
Response: While the Elixhauser Comorbidity Model is a good model which has been used for a long time, there are much more detailed data available in the electronic medical record of modern hospitals – data which were not available when scores like Elixhauser were developed. We set out to see how much we could improve the performance of Elixhauser – that is, its ability to predict outcomes such as mortality – by adding clinical data such as vital signs and laboratory values.
MedicalResearch.com: What are the main findings?
Response: The additional information created a model that is much, much more predictive. The difference in model performance is not subtle. The enhanced model we developed had much better ability to predict the outcomes of in-hospital mortality, need for intensive care, 30-day readmission, and length of hospital stay.
MedicalResearch.com: What should readers take away from your report?
Response: The additional information available in modern medical records allows real-time, automated calculation and display of patient risk scores that are much more predictive than the models available 20 years ago. Our enhanced risk adjustment model has several practical uses.
- First, the risk score could be automatically calculated and displayed to physicians in the emergency room, helping them to decide which patients require admission based on how high-risk they are.
- Second, the risk score could be used to adjust payments to hospitals, to take into account that hospitals treating sicker, more complex patient populations need to receive more money so they can hire more staff.
- Third, our model can be used to facilitate comparisons of hospitals on quality of care after adjusting for differences in patient case mix.
Nothing to disclose.
Citation:
Leibner, G., Katz, D.E., Esayag, Y. et al. Incorporating clinical and demographic data into the Elixhauser Comorbidity Model: deriving and validating an enhanced model in a tertiary hospital’s internal medicine department. BMC Health Serv Res 24, 1523 (2024). https://doi.org/10.1186/s12913-024-11663-z
———
The information on MedicalResearch.com is provided for educational purposes only, and is in no way intended to diagnose, cure, or treat any medical or other condition.
Some links may be sponsored. Products are not warranted or endorsed.
Always seek the advice of your physician or other qualified health and ask your doctor any questions you may have regarding a medical condition. In addition to all other limitations and disclaimers in this agreement, service provider and its third party providers disclaim any liability or loss in connection with the content provided on this website.
Last Updated on December 12, 2024 by Marie Benz MD FAAD