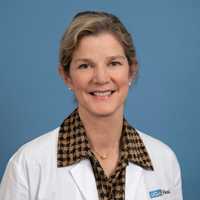
12 Aug AI Better At Reading Some Forms of Early Breast Cancer Pathology Slides
MedicalResearch.com Interview with:
Joann G. Elmore, MD, MPH
Professor of Medicine,
Director of the UCLA National Clinician Scholars Program
David Geffen School of Medicine at UCLA
MedicalResearch.com: What is the background for this study? What are the main findings?
- A pathologist makes the diagnosis of breast cancer versus non-cancer after reviewing the biopsy specimen. Breast biopsies are performed on millions of women each year and It is critical to get a correct diagnosis so that we can guide patients to the most effective treatments.
- Our prior work (Elmore et al. 2015 JAMA) found significant levels of disagreement among pathologists when they interpreted the same breast biopsy specimen. We also found that pathologists would disagree with their own interpretations of breast biopsies when they where shown the same biopsy specimen a year later.
- In this study, 240 breast biopsy images were fed into a computer, training it to recognize patterns associated with several types of breast lesions, ranging from benign (noncancerous) to invasive breast cancer. We compared the computer readings to independent diagnoses made by 87 practicing U.S. pathologists and found that while our artificial intelligence program came close to performing as well as human doctors in differentiating cancer from non-cancer, the AI program outperformed doctors when differentiating ductal carcinoma in situ (DCIS) from atypia, which is considered the greatest diagnostic challenge.
MedicalResearch.com: What should readers take away from your report?
- Future innovations in AI/machine learning show promise in aiding doctors to improve diagnostic performance and accuracy, especially for clinically challenging cases.
- This tool will help, not replace, pathologists by communicating what it “sees”. We have not yet reached a fully autonomous system that replaces the human element in health care delivery.
MedicalResearch.com: What recommendations do you have for future research as a result of this work?
Response: Further research is needed to understand the relationship of these findings with clinical outcomes and also with the decision-making process to enhance patient care.
MedicalResearch.com: Is there anything else you would like to add?
- Ezgi Mercan led this project as part of her PhD thesis work, under the supervision of Dr. Linda Shapiro, Professor of computer science at the University of WA. We thank Dr. Mercan for her invaluable scientific efforts on creating a tool and language describing cancerous structures. We also acknowledge Dr. Jamen Bartlett for taking on the laborious task of labeling images over several months, Dr. Donald Weaver for his clinical input and Sachin Mehta, a doctoral student at the University of Washington, who also made important contributions to the project. All of these contributions made it possible for the machine learning algorithms to perform as well as they did.
- This work was supported by a grant from the National Cancer Institute. Dr. Elmore currently serves as editor-in-chief for primary care topics at UpToDate.
Citation:
[wysija_form id=”3″]
[last-modified]
The information on MedicalResearch.com is provided for educational purposes only, and is in no way intended to diagnose, cure, or treat any medical or other condition. Always seek the advice of your physician or other qualified health and ask your doctor any questions you may have regarding a medical condition. In addition to all other limitations and disclaimers in this agreement, service provider and its third party providers disclaim any liability or loss in connection with the content provided on this website.
Last Updated on August 12, 2019 by Marie Benz MD FAAD