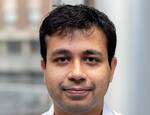
26 Feb SLIDE: Machine Learning Technique Can Infer Hidden States Underlying/Driving Disease Processes
MedicalResearch.com Interview with:
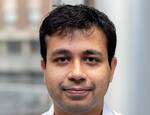
Dr. Das
Jishnu Das, Ph.D.
Center for Systems Immunology
Departments of Immunology and Computational & Systems Biology,
Assistant Professor School of Medicine
University of Pittsburgh
MedicalResearch.com: What is the background for this study? How does this new AI model work? How is it different from other models?
Response: Modern multi-omic technologies generate an enormous amount of data across scales of organization, and with differing resolution. While recent machine learning methods have harnessed these to predict clinical/physiological outcomes, they are often black boxes that do not provide meaningful inference beyond prediction. Differences in data generation modalities, redundancy in the data, as well as large numbers of irrelevant features make inference of biological mechanisms from high-dimensional omic datasets challenging.
To address these challenges, we developed a machine learning technique called SLIDE (Significant Latent Factor Interaction Discovery and Exploration). We reasoned that features that are directly measured by current technologies are constrained by strengths and weaknesses of current platforms. So, while some observed features may be excellent correlates of outcomes of interest, inferring biological mechanisms from these multi-omic datasets requires us to delve beyond the observable into the hidden states, i.e., latent factors. These hidden states encapsulate the true drivers of underlying biological processes and capture a complex multi-scale interplay between entities measured by these datasets. Our method moves beyond simple biomarkers/correlates (“the what”) to hidden states that actually explain clinical/physiological outcomes (“the how” and “the why”).
MedicalResearch.com: What types of disease processes may be better elucidated from SLIDE investigations?
Response: We used SLIDE to uncover drivers of a range of molecular, cellular and organismal (disease) phenotypes across contexts. For example, we uncovered a novel transcriptomic signature underlying pathogenesis of scleroderma, an autoimmune disorder that causes thickening of the skin and may damage other organs, involving cross-talk across multiple cell types. While prior work had uncovered biomarkers of scleroderma pathogenesis in myeloid cells and fibroblasts, SLIDE implicated a novel role for keratinocytes in scleroderma pathogenesis. It also helped “connect the dots” by explaining communication across these three cell types. More generally, because SLIDE helps infer the pathophysiology of disease (beyond just biomarkers), it can better help design interventional and therapeutic strategies.
MedicalResearch.com: What should readers take away from your report?
Response: SLIDE is a first-in-class interpretable machine learning approach that can infer hidden states underlying/driving biological and disease outcomes. While modern technologies offer us an opportunity to measure a very large number of quantities (would the word “variables” maybe be better than “quantities”?), answers to complex biological questions often necessitate looking beyond the observables. Our study also emphasizes the importance of moving beyond prediction (predictive machine learning) to inference (interpretable machine learning) as the latter is of vital importance in many biological contexts.
MedicalResearch.com: Is there anything else you would like to add? Any disclosures?
Response: This project reflects a true interdisciplinary collaboration between computational and experimental systems immunologists, clinicians and theoretical statisticians. The structure of the study is recursive — latent states reflecting the shared interests and expertise of overlapping author groups facilitated the formulation of this unique latent factor approach. Translating our different vocabularies and converging on a shared lexicon was both the most challenging and most rewarding part of this effort. This study reflects the possibilities that interdisciplinary science and “uncommon” interactions can offer.
I have no disclosures to report
Citation: Rahimikollu, J., Xiao, H., Rosengart, A. et al. SLIDE: Significant Latent Factor Interaction Discovery and Exploration across biological domains. Nat Methods (2024). https://doi.org/10.1038/s41592-024-02175-z
https://www.nature.com/articles/s41592-024-02175-z
The information on MedicalResearch.com is provided for educational purposes only, and is in no way intended to diagnose, cure, or treat any medical or other condition.
Some links may be sponsored. Products are not endorsed.
Always seek the advice of your physician or other qualified health and ask your doctor any questions you may have regarding a medical condition. In addition to all other limitations and disclaimers in this agreement, service provider and its third party providers disclaim any liability or loss in connection with the content provided on this website.
Last Updated on February 26, 2024 by Marie Benz MD FAAD