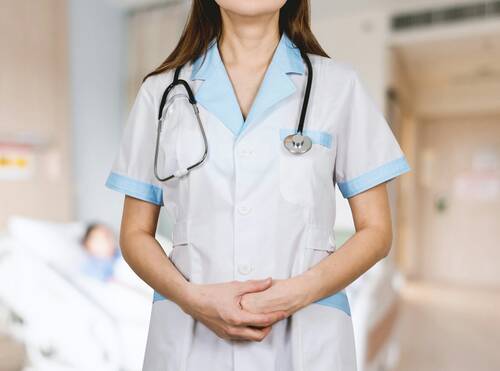
28 Feb Bridging the Gap Between Big Data and Healthcare: The Role of AI in Medical Research
The explosion of big data in healthcare has created an unprecedented opportunity to drive innovation in medical research, diagnostics, and treatment strategies. However, harnessing vast amounts of clinical, genomic, and patient-generated data presents significant challenges. Artificial Intelligence (AI) and machine learning (ML) are now at the forefront of transforming how researchers process, analyze, and apply this data to improve patient outcomes.
How AI is Unlocking the Power of Big Data in Medical Research
Historically, medical research relied on manual data collection and statistical models that often struggled to identify complex patterns in disease progression and treatment response. With the rise of AI-driven analytics, researchers can now extract valuable insights from massive, unstructured datasets in ways that were previously impossible.
Key advancements in AI-driven medical research include:
- Predictive Analytics for Disease Prevention – AI algorithms analyze electronic health records (EHRs) and genetic data to predict an individual’s likelihood of developing chronic diseases such as diabetes, cardiovascular conditions, and neurodegenerative disorders. These models can also identify previously unrecognized risk factors by cross-referencing genetic markers, lifestyle habits, and environmental exposures. By integrating AI-powered risk assessments into preventive healthcare strategies, physicians can offer personalized interventions before a disease manifests.
- Faster Drug Discovery and Development – AI models assist pharmaceutical companies in identifying promising drug candidates, reducing both costs and the time required for clinical trials. Traditional drug discovery processes take years to test and validate, but AI can analyze millions of molecular compounds in a fraction of the time. Additionally, AI-powered simulations enable researchers to predict drug efficacy and side effects, helping streamline the approval process.
- AI-Powered Medical Imaging – Machine learning is improving the accuracy of radiology, pathology, and MRI scans, enabling early detection of cancers and other critical conditions with unprecedented precision. AI-driven imaging tools are trained on vast datasets, allowing them to detect anomalies that even experienced radiologists might overlook. This not only enhances diagnostic accuracy but also enables real-time, automated image analysis for faster clinical decision-making.
Challenges in Integrating AI and Big Data in Medical Research
While AI has the potential to revolutionize medical research, challenges remain in data standardization, security, and ethical concerns. Medical datasets are often fragmented across hospitals, research institutions, and private organizations, making integration complex.
Another major challenge is the variability in data quality. AI algorithms require vast amounts of high-quality, structured data, but many healthcare records contain inconsistencies, missing values, or biases that can impact AI predictions. Furthermore, cybersecurity concerns arise as AI systems process sensitive patient information, necessitating stronger encryption, data governance frameworks, and compliance with regulations like HIPAA and GDPR.
Additionally, ensuring AI-driven models are free from bias is crucial to prevent disparities in healthcare outcomes. Regulatory bodies and research institutions are working to establish guidelines for ethical AI use, balancing innovation with patient privacy protections. Bias can emerge if training datasets lack diversity, leading to inaccuracies in medical diagnoses for underrepresented populations. Addressing this requires continuous model training, diverse datasets, and transparent AI governance.
The Future of AI in Healthcare Research
As AI technology continues to evolve, the integration of machine learning with genomic research, biometrics, and real-world evidence will drive even more significant breakthroughs in personalized medicine and public health strategies. AI is already being used to identify genetic mutations linked to rare diseases, allowing for more targeted therapies and early intervention strategies. In the near future, AI-driven tools may even personalize treatment recommendations based on real-time patient monitoring, optimizing care for individuals with chronic conditions.
Furthermore, machine learning enablement is empowering medical researchers with the tools needed to streamline data processing, enhance predictive modeling, and generate faster, more accurate insights. By integrating AI-driven automation into clinical trials, epidemiological research, and hospital operations, medical professionals can enhance efficiency while reducing human error. The adoption of AI in research also opens doors for real-time collaboration between institutions, enabling faster responses to global health threats such as pandemics.
Conclusion
The intersection of big data and AI is reshaping the future of medical research, offering new possibilities for disease prevention, early diagnosis, and personalized treatment. The sheer volume of medical data being generated daily makes AI-powered analytics an essential tool for researchers, clinicians, and pharmaceutical companies. While challenges remain in terms of data privacy, regulatory compliance, and bias reduction, the potential benefits far outweigh the risks.
By leveraging AI-driven analytics, medical research is moving towards a more predictive, precise, and patient-centered approach, one that will continue to revolutionize healthcare for years to come. As technology advances, the key to success lies in collaborations between AI developers, healthcare providers, and policymakers to ensure AI-driven solutions remain ethical, effective, and accessible to all patients.
More information:
—————
The information on MedicalResearch.com is provided for educational purposes only, and is in no way intended to diagnose, cure, or treat any medical or other condition.
Some links are sponsored. Products and services are not tested, warranted or endorsed.
Always seek the advice of your physician or other qualified health and ask your doctor any questions you may have regarding a medical condition. In addition to all other limitations and disclaimers in this agreement, service provider and its third party providers disclaim any liability or loss in connection with the content provided on this website.
Last Updated on February 28, 2025 by Marie Benz MD FAAD