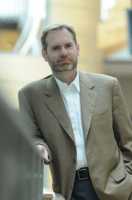
30 Apr Machine Learning Can Analyze Entire Transcriptome To Improve Diagnosis of Difficult Cancers
MedicalResearch.com Interview with:
Steven J.M. Jones, Professor, FRSC, FCAHS
Co-Director & Head, Bioinformatics
Genome Sciences Centre
British Columbia Cancer Research Centre
Vancouver, British Columbia, Canada and
Jasleen Grewal, BSc.
Genome Sciences Centre
British Columbia Cancer Research Centre
Vancouver, British Columbia, Canada
MedicalResearch.com: What is the background for this study?
Response: Cancer diagnosis requires manual analysis of tissue appearance, histology, and protein expression. However, there are certain types of cancers, known as cancers of unknown primary, that are difficult to diagnose based purely on their appearance and a small set of proteins. In our precision medicine oncogenomics program, we needed an accurate approach to confirm diagnosis of biopsied samples and determine candidate tumour types for where the primary site of the cancer was uncertain. We developed a machine learning approach, trained on the gene expression data of over 10,688 individual tumours and healthy tissues, that has been able to achieve this task with high accuracy.
Genome sequencing offers a high-resolution view of the biological landscape of cancers. RNA-Seq in particular quantifies how much each gene is expressed in a given sample. In this study, we used the entire transcriptome, spanning 17,688 genes in the human genome, to train a machine learning method for cancer diagnosis. The resultant method, SCOPE, takes in the entire transcriptome and outputs an interpretable confidence score from across a set of 40 different cancer types and 26 healthy tissues.
MedicalResearch.com: What are the main findings?
Response: We found that the method had ~99% accuracy in identifying cancers with mixed tissue types, and had a success rate of 80-86% in the most challenging cases that had already failed human assessment or were extremely difficult to diagnose by a human expert (cancers of unknown origin and advanced cancers).
MedicalResearch.com: What should readers take away from your report?
Response: Using the entire transcriptome for cancer diagnosis coupled with machine learning is a novel approach for cancer diagnosis. It assesses the gene expression of all protein-coding genes to provide a confidence score, making the predictions interpretable and reliable. In particular, it is a powerful orthogonal diagnostic in cases where the diagnosis cannot be determined through pathology. In maybe a more controversial interpretation, it also highlights the progress machine learning approaches have made in fields previously considered to be the domain of highly skilled human expertise and demonstrates where computational approaches can not only augment but improve upon clinical decision making.
MedicalResearch.com: What recommendations do you have for future research as a result of this work?
Response: Machine learning methods are only as good as the data they have to train on. Efforts are needed to properly curate and sequence rare and advanced cancers so that we can better incorporate them in such models, ultimately improving our ability to identify and diagnose them. Future research should also examine the ability to leverage the entirety of sequencing data for other manually-driven cancer analysis tasks, such as the alignment with appropriate therapies. But more interestingly will be the potential to dissect what this artificial intelligence approach has learnt about cancer and whether it has been able to determine subtleties and facets about the disease that have been eluding us.
MedicalResearch.com: Is there anything else you would like to add?
Response: This study highlights a novel quantitative approach for cancer diagnosis – using the entirety of RNA-Seq data instead of relying purely on expert manual assessment or limited gene panels. As shown by our findings, there is huge potential to develop interpretable machine-learning methods using the entirety of sequencing data. Algorithms that incorporate this high-resolution data as a whole to provide insights into cancers can serve as a powerful means of analysis and decision-making.
Citation:
[wysija_form id=”3″]
[last-modified]
The information on MedicalResearch.com is provided for educational purposes only, and is in no way intended to diagnose, cure, or treat any medical or other condition. Always seek the advice of your physician or other qualified health and ask your doctor any questions you may have regarding a medical condition. In addition to all other limitations and disclaimers in this agreement, service provider and its third party providers disclaim any liability or loss in connection with the content provided on this website.
Last Updated on April 30, 2019 by Marie Benz MD FAAD