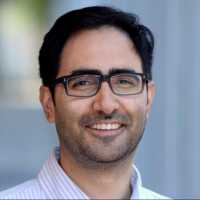
15 Jul Janggu Technology Enhances Deep Learning For Genomics
MedicalResearch.com Interview with:
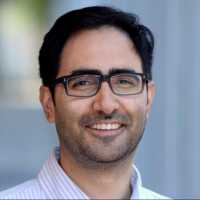
Dr. Akalin
Dr.Altuna Akalin PhD
Head of Bioinformatics and Omics Data Science Platform
Berlin Institute for Medical Systems Biology (BIMSB)
Max Delbrück Center for Molecular Medicine (MDC)
Berlin, Germany
MedicalResearch.com: What is the background for this study? Where does the word Janggu come from?
Response: Deep learning applications on genomic datasets used to be a cumbersome process where researchers spend a lot of time on preparing and formatting data before they even can run deep learning models. In addition, the evaluation of deep learning models and the choice of deep learning framework were also not straightforward. To streamline these processes, we developed Janggu. With this framework, we are aiming to relieve some of that technical burden and make deep learning accessible to as many people as possible.
Janggu is named after a traditional Korean drum shaped like an hourglass turned on its side. The two large sections of the hourglass represent the areas Janggu is focused: pre-processing of genomics data, results visualization and model evaluation. The narrow connector in the middle represents a placeholder for any type of deep learning model researchers wish to use.
MedicalResearch.com: What are the main findings? What are some of the potential applications?
Response: We have developed a deep learning based tool for genomic datasets. What makes our approach special is that you can easily use any genomic data set for your deep learning problem, it can be genome sequences or high-throughput sequencing data such as RNA-seq or ATAC-seq, anything goes in any format. There is also no limitation in which deep learning library you will use, most popular libraries, and also standard machine learning libraries are supported.
The applications of this approach are endless. We can predict any feature in the genome given relevant data sets for that feature. One of the most interesting applications is predicting the effect of mutations on gene regulation. This is exciting because now we can start understanding individual genomes, for instance, we can pinpoint genetic variants that cause regulatory changes or we can interpret regulatory mutations occurring in tumors.
Above all, the only thing users need to get started with deep learning is their data and their regions of interest. Our approach will take care of normalization, data wrangling and predictive performance evaluation so that researchers spend more time tuning their deep learning models rather than dealing with technicalities.
MedicalResearch.com: What should readers take away from your report?
Response: Deep learning applications on genomics are now easier than ever. We streamline the most cumbersome tasks so this technology is more accessible to more researchers.
We would like to thank all our collaborators on this paper and users who gave us feedback so that we can improve Janggu.
Citation:
Wolfgang Kopp, Remo Monti, Annalaura Tamburrini, Uwe Ohler, Altuna Akalin. Deep learning for genomics using Janggu. Nature Communications, 2020; 11 (1) DOI: 10.1038/s41467-020-17155-y
[subscribe]
[last-modified]
The information on MedicalResearch.com is provided for educational purposes only, and is in no way intended to diagnose, cure, or treat any medical or other condition. Always seek the advice of your physician or other qualified health and ask your doctor any questions you may have regarding a medical condition. In addition to all other limitations and disclaimers in this agreement, service provider and its third party providers disclaim any liability or loss in connection with the content provided on this website.
Last Updated on July 15, 2020 by Marie Benz MD FAAD