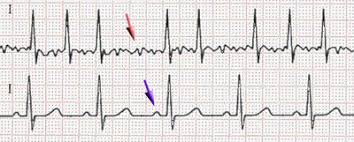
02 Aug AI-Trained EKG Can Detect New or Impending Atrial Fibrillation
MedicalResearch.com Interview with:
Paul Friedman, M.D.
Professor of Medicine
Norman Blane & Billie Jean Harty Chair
Mayo Clinic Department of Cardiovascular Medicine
Honoring Robert L. Frye, M.D.
MedicalResearch.com: What is the background for this study?
Response: Atrial fibrillation is an irregular heart rhythm that is often intermittent and asymptomatic. It is estimated to affect 2.7–6.1 million people in the United States, and is associated with increased risk of stroke, heart failure and mortality. It is difficult to detect and often goes undiagnosed. After an unexplained stroke, it is important to accurately detect atrial fibrillation so that patients with it are given anticoagulation treatment to reduce the risk of recurring stroke, and other patients (who may be harmed by this treatment) are not. Currently, detection in this situation requires monitoring for weeks to years, sometimes with an implanted device, potentially leaving patients at risk of recurrent stroke as current methods do not always accurately detect atrial fibrillation, or take too long.
We hypothesized that we could train a neural network to identify the subtle findings present in a standard 12-lead electrocardiogram (ECG) acquired during normal sinus rhythm that are due to structural changes associated with a history of (or impending) atrial fibrillation. Such an AI enhanced ECG (AI ECG) would be inexpensive, widely available, noninvasive, performed in 10 seconds, and immensely useful following embolic stroke of unknown source to guide therapy. To test this hypothesis, we trained, validated, and tested a deep convolutional neural network using a large cohort of patients from the Mayo Clinic Digital Data Vault.
MedicalResearch.com: What are the main findings?
Response: We found that the AI ECG powerful in its ability to detect recent or impending atrial fibrillation, with an AUC of 0.90. This compares favorably with other medical screening tests such as B-type natriuretic peptide for heart failure (AUC 0·60–0·70), Papanicolaou smear for cervical cancer (AUC 0·70), and the CHA2DS2-VASc Score for stroke risk
(AUC 0·57–0·72).
Additionally, once a network is trained, it can be applied to any standard digital 12-lead ECG with minimal computing power requirements; for example, a smartphone could process the signals. In the future, this might facilitate point-of-care diagnosis by allowing application of the algorithm on low-cost, widely available technologies. For instance, we have previously shown the translation of neural networks created using 12-lead ECGs to mobile, smartphone-based electrodes that typically include a single lead.
MedicalResearch.com: What should readers take away from your report?
Response: The addition of AI to a standard, widely available, inexpensive noninvasive test transforms it into a powerful that can detect recent or impending atrial fibrillation, which may be useful to guide treatment to prevent strokes, heart failure and death. It does this due to the ability of the network to see subtle patterns in the network that are hidden in plain sight.
MedicalResearch.com: What recommendations do you have for future research as a result of this work?
Response: Future studies will prospectively test treatment to prevent stroke on the basis of the AI ECG, avoiding the need for procedures to implant heart rhythm monitoring devices and years of monitoring.
No disclosures
Citation:
An artificial intelligence-enabled ECG algorithm for the identification of patients with atrial fibrillation during sinus rhythm: a retrospective analysis of outcome prediction
Attia, Zachi I et al.
The Lancet, Volume 0, Issue 0
https://www.thelancet.com/journals/lancet/article/PIIS0140-6736(19)31721-0/fulltext
[wysija_form id=”3″]
[last-modified]
The information on MedicalResearch.com is provided for educational purposes only, and is in no way intended to diagnose, cure, or treat any medical or other condition. Always seek the advice of your physician or other qualified health and ask your doctor any questions you may have regarding a medical condition. In addition to all other limitations and disclaimers in this agreement, service provider and its third party providers disclaim any liability or loss in connection with the content provided on this website.
Last Updated on August 2, 2019 by Marie Benz MD FAAD