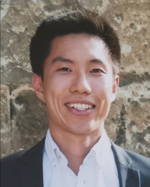
11 Nov Dermatology: Datasets Used for AI Lack Diversity and Completeness
MedicalResearch.com Interview with:
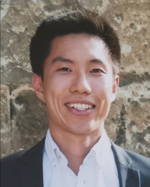
Dr. Wen
Dr David Wen BM BCh
NIHR Academic Clinical Fellow in Dermatology
University of Oxford
MedicalResearch.com: What is the background for this study?
Response: Publicly available skin image datasets are commonly used to develop machine learning (ML) algorithms for skin cancer diagnosis. These datasets are often utilised as they circumvent many of the barriers associated with large scale skin lesion image acquisition. Furthermore, publicly available datasets can be used as a benchmark for direct comparison of algorithm performance.
Dataset and image metadata provide information about the disease and population upon which the algorithm was trained or validated on. This is important to know because machine learning algorithms heavily depend on the data used to train them; algorithms used for skin lesion classification frequently underperform when tested on independent datasets to which they were trained on. Detailing dataset composition is essential for extrapolating assumptions of generalisability of algorithm performance to other populations.
At the time this review was conducted, the total number of publicly available datasets globally and their respective content had not previously been characterised. Therefore, we aimed to identify publicly available skin image datasets used to develop ML algorithms for skin cancer diagnosis, to categorise their data access requirements, and to systematically evaluate their characteristics including associated metadata.
MedicalResearch.com: What are the main findings?
Response: We identified 21 open access datasets containing 106,950 freely available images, and eight regulated access datasets which required payment or formal approvals to access in full.
We reviewed the open access datasets in further detail. With regards to their general characteristics:
- Fourteen of 21 datasets reported which country they originated from and of those, eleven contained images from Europe, North America or Oceania only.
- Nineteen of 21 datasets contained images from one modality only (either macroscopic photographs or dermoscopic images – pictures taken with a special hand-held magnifier). Only two of the 21 datasets included images taken with both of these methods, which better reflects how dermatologists examine lesions in clinical practice.
- Many datasets were also missing other important information, such as how images were chosen to be included, and evidence of ethical approval or patient consent.
Regarding metadata reporting for individual images in the open access datasets:
- Approximately 75% of individual images had metadata labels for age, sex and lesion site.
- Only 2% of individual images had metadata labels for skin type, and only 1% for ethnicity.
- Of the 2,436 images from three datasets where skin type information was available, ten images were from subjects with Fitzpatrick type V (brown) skin, and one image was from an individual with Fitzpatrick type VI (dark brown or black) skin.
- Of the 1,585 images from two datasets where ethnicity was available, no images were from individuals with an African, Afro-Caribbean or South Asian background.
MedicalResearch.com: What recommendations do you have for future research as a result of this work?
Response: Our review highlights that better reporting of dataset characteristics and metadata is required with the aim of producing transparent datasets. Quality standards outlining what should be reported in datasets may facilitate this through providing guidance for dataset curators.
Datasets should be representative of the target populations that any developed algorithms will be deployed in. Dataset standards can also detail what constitutes a representative dataset. To ensure inclusion of all groups, images may need to be collected prospectively (going forward in time), rather than retrospectively (selecting images which have already been taken, for example as part of clinical care) which can be susceptible to selection bias.
MedicalResearch.com: Is there anything else you would like to add?
Response: This study is independent research funded by NHSX and the Health Foundation. Four authors reported being paid employees of Databiology at the time of the study. The other authors reported no relevant financial relationships.
Citation:
Characteristics of publicly available skin cancer image datasets: a systematic review
Wen, David et al.
The Lancet Digital Health, Volume 0, Issue 0
https://www.thelancet.com/journals/landig/article/PIIS2589-7500(21)00252-1/fulltext
JOIN OUR EMAIL LIST
[mailpoet_form id="5"]We respect your privacy and will never share your details.
[last-modified]
The information on MedicalResearch.com is provided for educational purposes only, and is in no way intended to diagnose, cure, or treat any medical or other condition. Always seek the advice of your physician or other qualified health and ask your doctor any questions you may have regarding a medical condition. In addition to all other limitations and disclaimers in this agreement, service provider and its third party providers disclaim any liability or loss in connection with the content provided on this website.
Last Updated on November 11, 2021 by Marie Benz MD FAAD