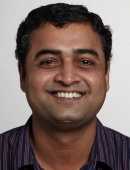
12 Feb Radiomics Plus Machine Learning Can Optimize Prostate Cancer Classification
MedicalResearch.com Interview with:
Gaurav Pandey, Ph.D.
Assistant Professor
Department of Genetics and Genomic Sciences
Icahn Institute of Data Science and Genomic Technology
Icahn School of Medicine at Mount Sinai, New York
MedicalResearch.com: What is the background for this study?
Response: Multiparametric magnetic resonance imaging (mpMRI) has become increasingly important for the clinical assessment of prostate cancer (PCa), most routinely through PI-RADS v2, but its interpretation is generally variable due to its relatively subjective nature.
Radiomics, a methodology that can analyze a large number of features of images that are difficult to study solely by visual assessment, combined with machine learning methods have shown potential for improving the accuracy and objectivity of mpMRI-based prostate cancer assessment. However, previous studies in this direction are generally limited to a small number of classification methods, evaluation using the AUC score only, and a non-rigorous assessment of all possible combinations of radiomics and machine learning methods.
MedicalResearch.com: What are the main findings?
Response: We developed a systematic and rigorous ML-based framework for deriving a reliable and objective prostate cancer risk classifier by finding the optimal classification method(s) for radiomic features derived from a given set of mpMRI imaging data. This framework was used to develop a radiomics-based classifier operating on mpMRI images of prostate cancer tumors that accurately distinguishes between subjects with high-risk prostate cancer and those with lower-risk PCa. Our classifier, built on top of 110 radiomic features interpreted via a Quadratic kernel-based Support Vector Machine (QSVM), performed with reasonably high precision or predictive value and high recall or sensitivity for classifying PCa patients in an independent validation set. This effectiveness of QSVM can be partly attributed to the use of kernels that can represent non-Euclidean similarity or distance between data points without having to transform the points into the non-Euclidean space. This classifier performed better than PI-RADS v2 on the same validation set, especially in terms of the class-specific evaluation measures indicating the value of objective assessment of prostate cancer risk as compared to a more subjective one.
MedicalResearch.com: What should readers take away from your report?
Response: The goal of combining machine learning and radiomics is not to compete with the radiologist, but to rather provide the radiologist and physician team taking care of the patient with objective prediction tools that can aid personalized decision making regarding individual disease course and treatment outcome. The use of such decision support systems will enhance the quality of the radiologist’s work and help in the long-run aid in the integration of such systems into routine patient care. We would also like to emphasize that, although the machine learning-based classifier derivation process may seem involved, the clinical practitioners don’t have to deal with it directly. The resultant classifier, which can typically be implemented in a few lines of code on top of the existing radiomics pipeline, can be executed by such practitioners through a simple GUI and only a few clicks of a mouse or key.
MedicalResearch.com: What recommendations do you have for future research as a result of this work?
Response: Systematic integration of quantitative disciplines like radiomics and machine learning with clinical data and practice have the potential to improve and personalize patient care, as we demonstrated in our validation study, and perhaps even transform it. Thus, our work is a call for more such cross-disciplinary integrations, as long as they are carried out systematically and rigorously. For instance, the application of machine learning is expected to be productive as long as care is taken to address common challenges like utilizing as large study cohorts as possible, avoiding sample overfitting and validation in terms of appropriate evaluation measures, like Precision, Recall and F-measure, rather than those routinely used in the community, like the AUC score.
Disclosures: This work was funded by the Big Data to Knowledge (BD2K) Training Coordinating Center (TCC) through the Data Science Rotations for Advancing Discovery (RoAD-Trip) program (NIH grant #1U24ES026465-03), NIH grant #R01GM114434, an IBM faculty award and grant #PC140371 from the Department of Defense Prostate Cancer Research Program. None of the funding agencies influenced the study’s conduct and outcomes in any way.
Citation:
Bino Varghese, Frank Chen, Darryl Hwang, Suzanne L Palmer, Andre Luis De Castro Abreu, Osamu Ukimura, Monish Aron, Manju Aron, Inderbir Gill, Vinay Duddalwar, Gaurav Pandey. Objective risk stratification of prostate cancer using machine learning and radiomics applied to multiparametric magnetic resonance images. Scientific Reports, 2019; 9 (1) DOI: 10.1038/s41598-018-38381-x
[wysija_form id=”3″]
[last-modified]
The information on MedicalResearch.com is provided for educational purposes only, and is in no way intended to diagnose, cure, or treat any medical or other condition. Always seek the advice of your physician or other qualified health and ask your doctor any questions you may have regarding a medical condition. In addition to all other limitations and disclaimers in this agreement, service provider and its third party providers disclaim any liability or loss in connection with the content provided on this website.
Last Updated on February 12, 2019 by Marie Benz MD FAAD