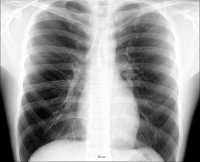
11 Dec AI and Chest X Rays: Google Health Algorithms Performed As Well As Radiologists
MedicalResearch.com Interview with:
Dr. David Steiner, MD PhD
Google Health, USA
MedicalResearch.com: What is the background for this study?
Response: Advances in artificial intelligence raise promising opportunities for improved interpretation of chest X-rays and many other types of medical images. However, even before researchers begin to address the critical question of clinical validation, there is important work to be done establishing strategies for evaluating and comparing different artificial intelligence algorithms.
One challenge is defining and collecting the correct clinical interpretation or “label” for the large number of chest X-rays needed to train and evaluate these algorithms. Another important challenge is evaluating the algorithm on a dataset that actually represents the diversity of the cases encountered in clinical practice. For example, it might be relatively easy to make an algorithm that performs perfectly on a few hundred or so “easy” cases, but this of course might not be particularly useful in practice.
MedicalResearch.com: What are the main findings?
Response: In our study, we used two large, independent datasets to develop algorithms to detect four important chest X-ray findings. These models performed as well as radiologists on average across thousands of diverse chest X-rays.
MedicalResearch.com: What should readers take away from your report?
Response: We were able to develop algorithms for chest X-ray interpretation that performed similarly on average to radiologists for four clinically important chest X-ray findings. Notably, evaluation of our algorithms involved a rigorous evaluation strategy that used a panel of radiologists to determine the correct ground-truth across a large and diverse set of X-rays. We are also pleased to be able to share thousands of the expert panel-based ground-truth labels that we used for evaluation so that other researchers can also develop, evaluate, and compare efforts with this resource.
MedicalResearch.com: What recommendations do you have for future research as a result of this work?
Response: We hope this work helps demonstrate the importance of evaluating algorithms on diverse datasets, using rigorous ground truth labels, and paying attention to whether or not the images used for evaluation reflect an appropriately representative mix of cases. With this as an important benchmark for evaluation, understanding how such algorithms perform in different clinical settings and on different patient populations remains an important question. Future work performing detailed analyses on the specific strengths and weaknesses of algorithms will also be important and valuable. Ultimately, creating actual clinical applications for AI systems will require thoughtful workflow integration and studies to understand the impact of such systems on clinical practice and patient outcomes.
For additional information, you can also see our research blog post about this work.
Citation: RSNA 2019 abstract
Anna Majkowska, Sid Mittal, David F. Steiner, Joshua J. Reicher, Scott Mayer McKinney, Gavin E. Duggan, Krish Eswaran, Po-Hsuan Cameron Chen, Yun Liu, Sreenivasa Raju Kalidindi, Alexander Ding, Greg S. Corrado, Daniel Tse, and Shravya Shetty
Radiology 0 0:0
[subscribe]
Last Modified: [last-modified]
The information on MedicalResearch.com is provided for educational purposes only, and is in no way intended to diagnose, cure, or treat any medical or other condition. Always seek the advice of your physician or other qualified health and ask your doctor any questions you may have regarding a medical condition. In addition to all other limitations and disclaimers in this agreement, service provider and its third party providers disclaim any liability or loss in connection with the content provided on this website.
Last Updated on December 11, 2019 by Marie Benz MD FAAD