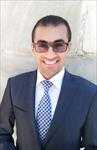
23 Nov Deep Learning of Germline Mutations Enables Identification of More Patients at Risk of Cancer
MedicalResearch.com Interview with:
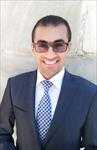
Dr. AlDubayan
Saud H AlDubayan, M.D.
Instructor in Medicine, Harvard Medical School
Attending Physician, Division of Genetics,
Brigham and Women’s Hospital
Computational Biologist, Department of Medical Oncology,
Dana-Farber Cancer Institute
Associate Scientist, The Broad Institute of MIT and Harvard
MedicalResearch.com: What is the background for this study? What are the main findings?
Response: The overall goal of this study was to assess the performance of the standard method currently used to detect germline (inhered) genetic variants in cancer patients and whether we could use recent advances in machine learning techniques to further improve the detection rate of clinically relevant genetic alterations.
To investigate this possibility, we performed a head to head comparison between the current gold-standard method for germline analysis that has been universally used in clinical and research laboratories and a new deep learning analysis approach using germline genetic data of thousands of patients with prostate cancer or melanoma. This analysis showed that across all different gene sets that were tested, the deep learning-based framework was able to identify additional cancer patients with clinically relevant germline variants that went undetected by the standard method. For example, several patients in our study also had germline variants that are associated with an increased risk of ovarian cancer, for which the surgical removal of the ovaries (at a certain age) is highly recommended. However, these genetic alterations were only identified by the proposed deep learning framework.
MedicalResearch.com: What should readers take away from your report?
Response: Overall, this study demonstrates the potential to improve the diagnostic yield of germline genetic testing, which has become an integral part of the clinical management of cancer patients. For example, germline testing can identify patients and families who have an inherited higher risk for developing various types of cancer in whom a more enhanced and personalized screening approach can be effective and lifesaving. Germline testing can also guide the therapeutic management of cancer patients since carriers of certain germline variants have greater response and benefit to targeted treatments. By improving the ability to identify clinically relevant germline alterations in cancer, we have extended the established clinical benefit of preventive and therapeutic interventions to additional cancer patients who would not otherwise be eligible for these interventions using the current analysis method.
MedicalResearch.com: What recommendations do you have for future research as a result of this study?
Response: Although deep learning was significantly more likely to identify cancer patients with clinically informative germline genetic alterations, deep learning did not detect all genetic alterations present in the examined cancer patient cohorts. This highlights an opportunity for future research to build on these advances to further improve the performance of germline genetic testing and accelerate gene discovery in this field.
No disclosures of conflict of interest to declare.
Citation:
AlDubayan SH, Conway JR, Camp SY, et al. Detection of Pathogenic Variants With Germline Genetic Testing Using Deep Learning vs Standard Methods in Patients With Prostate Cancer and Melanoma. JAMA. 2020;324(19):1957–1969. doi:10.1001/jama.2020.20457
The information on MedicalResearch.com is provided for educational purposes only, and is in no way intended to diagnose, cure, or treat any medical or other condition. Always seek the advice of your physician or other qualified health and ask your doctor any questions you may have regarding a medical condition. In addition to all other limitations and disclaimers in this agreement, service provider and its third party providers disclaim any liability or loss in connection with the content provided on this website.
Last Updated on November 23, 2020 by Marie Benz MD FAAD