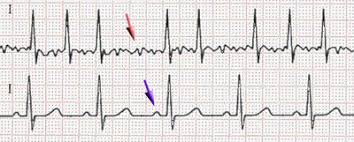
12 Mar Stroke Risk: AI Can Help Predict Who Might Develop Atrial Fibrillation
MedicalResearch.com Interview with:
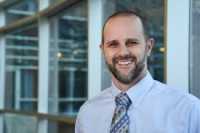
Dr. Fornwalt
Brandon K Fornwalt, MD, PhD
Associate Professor, Director Department of Imaging Science and Innovation
Geisinger
MedicalResearch.com: What is the background for this study?
Response: Atrial fibrillation (AF) is an abnormal heart rhythm that is associated with outcomes such as stroke, heart failure and death. If we know a patient has atrial fibrillation, we can treat them to reduce the risk of stroke by nearly two-thirds. Unfortunately, patients often don’t know they have AF. They present initially with a stroke, and we have no chance to treat them before this happens. If we could predict who is at high risk of either currently having AF or developing it in the near future, we could intervene earlier and hopefully reduce bad outcomes like stroke. Artificial intelligence approaches may be able to help with this task.
MedicalResearch.com: What are the main findings? Is the technology to do this calculation widely available?
Response: We found that an artificial intelligence algorithm could automatically interpret data from a common medical test called an electrocardiogram and use this data to predict which patients were at high risk for developing AF in the next year. Moreover, on a large dataset of 1.6M electrocardiograms collected from patients at Geisinger over the last several decades, we showed the model predicted 62% of all patients who experienced an AF-related stroke as high risk, before the stroke happened. This suggests earlier intervention could potentially avoid such strokes. Currently, this algorithm is only available at Geisinger, and we are working on ways to make this algorithm more widely available.
MedicalResearch.com: What should readers take away from your report?
Response: Artificial intelligence can help assist clinicians to better predict important future clinical events before they happen, enabling earlier diagnosis and treatment to hopefully improve patient outcomes. We showed feasibility of this in the case of AF-associated stroke, but expect this to be true of many other diseases.
MedicalResearch.com: What recommendations do you have for future research as a result of this work?
Response: We are working on designing and launching a prospective trial to help demonstrate that using this new technology has a positive impact on patients.
MedicalResearch.com: Is there anything else you would like to add?
Response: There is a lot of excitement about artificial intelligence changing medicine, but we need more practical examples of exactly how this will benefit patients. We are excited about this work representing a real-world example of the potential to use AI to transform the way we care for patients and improve their lives.
Citation:
Disclosures are listed in the publication available at: https://www.ahajournals.org/doi/10.1161/CIRCULATIONAHA.120.047829
Deep Neural Networks Can Predict New-Onset Atrial Fibrillation From the 12-Lead Electrocardiogram and Help Identify Those at Risk of AF-Related Stroke
Sushravya Raghunath , John M. Pfeifer , Alvaro E. Ulloa-Cerna , Arun Nemani , Tanner Carbonati , Linyuan Jing , David P. vanMaanen , Dustin N. Hartzel , Jeffrey A. Ruhl , Braxton F. Lagerman , Daniel B. Rocha , Nathan J. Stoudt , Gargi Schneider , Kipp W. Johnson , Noah Zimmerman , Joseph B. Leader , H. Lester Kirchner , Christoph J. Griessenauer , Ashraf Hafez , Christopher W. Good , Brandon K. Fornwalt , and Christopher M. Haggerty
[subscribe]
[last-modified]
The information on MedicalResearch.com is provided for educational purposes only, and is in no way intended to diagnose, cure, or treat any medical or other condition. Always seek the advice of your physician or other qualified health and ask your doctor any questions you may have regarding a medical condition. In addition to all other limitations and disclaimers in this agreement, service provider and its third party providers disclaim any liability or loss in connection with the content provided on this website.
Last Updated on March 12, 2021 by Marie Benz MD FAAD