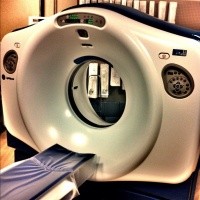
16 Oct AI: Deep Learning Algorithms Can Detect Critical Head CT Findings
MedicalResearch.com Interview with:
Sasank Chilamkurthy
AI Scientist,
Qure.ai
MedicalResearch.com: What is the background for this study?
Response: Head CT scan is one of the most commonly used imaging protocols besides chest x-ray. They are used for patients with symptoms suggesting stroke, rise in intracranial pressure or head trauma. These manifest in findings like intracranial haemorrhage, midline shift or fracture.
Scans with these critical findings need to be read immediately. But radiologists evaluate the scans on first-come-first-serve basis or based on stat/routine markers set by clinicians. If the scans with critical findings are somehow pushed to the top of radiologists’ work list, it could substantially decrease time to diagnosis and therefore decrease mortality and morbidity associated with stroke/head trauma.
MedicalResearch.com: What are the main methods and findings?
Response: Artificial intelligence (AI) technique called deep learning has been used recently to detect abnormalities on imaging techniques like fundus photography, chest x-ray and chest CT. In the same vein, we used deep learning to create algorithms which can detect critical abnormalities like haemorrhages or midline shift from head CT scans.
We have validated these algorithms versus radiologists’ opinion on two datasets (n=20195 and 491 respectively). Original radiology report and majority opinion of three independent radiologists were considered gold standards for first and second datasets respectively. Algorithms achieved Areas Under the Curve (AUCs) greater than 0.90 for 9 different findings which require urgent attention.
MedicalResearch.com: What should readers take away from your report?
Response: Deep learning algorithms can accurately identify scans requiring immediate attention. These algorithms can be used to pre-read head CT scans as they are acquired and triage them in order of criticality. They could also provide a lower performance bound for quality and consistency of radiological interpretation.
MedicalResearch.com: What recommendations do you have for future research as a result of this work?
Response: Now that the AI algorithms showed promising results on retrospective data, they need to be prospectively validated in-vivo. Triage using AI algorithms might improve radiologist efficiency but also has potential to introduce biases in radiologists. A clinical trial can be used to determine the safety and efficacy of such a triage.
MedicalResearch.com: Is there anything else you would like to add?
Response: This study was funded by Qure.ai and done in collaboration with Centre for advanced research in imaging, neurosciences and genomics and researchers from Mayo clinic. In addition to the above head CT algorithms, Qure.ai develops deep learning algorithms for detecting various abnormalities on chest X-rays. A validation study of qure.ai’s chest X-ray algorithms, done in collaboration with researchers from Massachusetts General Hospital, was also published recently in PLOS One.
Citation:
Deep learning algorithms for detection of critical findings in head CT scans: a retrospective study
Sasank Chilamkurthy, BTech Rohit Ghosh, BTech Swetha Tanamala, MTech Mustafa Biviji, DNB Norbert G Campeau, MD Vasantha Kumar Venugopal, MD et al.
Published:October 11, 2018DOI:https://doi.org/10.1016/S0140-6736(18)31645-3
[wysija_form id=”3″]
[last-modified]
The information on MedicalResearch.com is provided for educational purposes only, and is in no way intended to diagnose, cure, or treat any medical or other condition. Always seek the advice of your physician or other qualified health and ask your doctor any questions you may have regarding a medical condition. In addition to all other limitations and disclaimers in this agreement, service provider and its third party providers disclaim any liability or loss in connection with the content provided on this website.
Last Updated on October 16, 2018 by Marie Benz MD FAAD