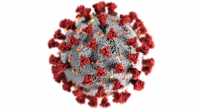
02 Apr Data-Driven AI May Help Predict Severity of Coronavirus
MedicalResearch.com Interview with:
Professor Anasse Bari PhD
Courant Institute of Mathematical Sciences,
Computer Science Department, New York University, New York, and
Megan Coffee MD PhD
Division of Infectious Diseases and Immunology, Department of Medicine
New York University,
Department of Population and Family Health
Mailman School of Public Health
Columbia University, New York
MedicalResearch.com: What is the background for this study?
Coffee and Bari: This work is led by NYU Grossman School of Medicine and NYU’s Courant Institute of Mathematical Sciences, in partnership with Wenzhou Central Hospital and Cangnan People’s Hospital, both in Wenzhou, China. This is a multi-disciplinary team with backgrounds in clinical infectious disease as well as artificial intelligence (AI) and computer science.
There is a critical need to better understand COVID-19. Doctors learn from collective and individual clinical experiences. Here, no clinician has years of experience. All are learning as they go, having to make important decisions about clinical management with stretched resources. The goal here is to augment clinical learning with machine learning.
In particular, the goal is to allow clinicians to identify early who from the many infected will need close medical attention. Most patients will first develop mild symptoms, yet some 5-8 days later will develop critical illness. It is hard to know who these people are who will need to be admitted and may need to be intubated until they become ill. Knowing this earlier would allow more attention and resources to be spent on those patients with worse prognoses. If there were ever treatments in the future that could be used early in the course of illness, it would be important to identify who would most benefit
We present in this study a first step in building an artificial intelligence (AI) framework, with predictive analytics (PA) capabilities applied to real patient data, to provide rapid clinical decision-making support. It is at this point a proof of concept that it could be possible to identify future severity based on initial presentation in COVID-19.
MedicalResearch.com: What are the main findings?
Response: Clinical data from a line listing was used to predict risk of ARDS. Although there were only 53 patients (all of whom have now been discharged), we were able to develop a model that can learn from this data.
AI often identifies features a doctor would not; ALT, myalgias, and hemoglobin all stood out as the most predictive. The findings are dependent on the data from our colleagues in Wenzhou, China where the case severity was less than is being seen now in New York City. We are at very early stages of developing this framework, we still need to do further do more validation and we need large datasets. We are now looking into expanding the work with New York hospitals and medical institutions around the world.
MedicalResearch.com: What should readers take away from your report?
Coffee and Bari: First, it should be noted that this model needs to be validated in different contexts before it could be used clinically, but we think this is a first step to using AI when the clinical workload is immense. AI is increasingly able to act as an extension to human intelligence. The experimental tool we developed, once deployed, could serve an extension to the work being done by doctors, to help them know early which cases will need more attention and resources. The tool will help doctors make data-driven decisions. We are not trying by any mean to replace doctors. Instead, we are tying to relieve doctors who have high caseloads and limited resources by identifying those patients that need them the most. Instead of scanning tables of data, the algorithm can help go through the data using learning from historical data, to identify which patients need the most clinical attention. In the end, though, the doctor must make the final decisions. Like any other AI, there is a continuous process of learning; doctors and new data sets will help correct and re-train the AI.
At this point the model points to clinical features a doctor might not consider as important. That’s what’s interesting about machine learning; it acts as a second opinion, so to speak. Small increases in liver tests and hemoglobin (red blood cells) appeared to predict severe disease more than other expected characteristics like age and gender. Moreover, characteristics that help diagnose coronavirus – low white blood cell count, chest imaging findings, fevers – did not appear to correspond to more severe disease.
MedicalResearch.com: What recommendations do you have for future research as a result of this work?
Coffee and Bari: It will be important that any tools using Artificial Intelligence are not black boxes. Clinicians need to be involved throughout the process so that any tools developed are clinically relevant. We used ARDS (acute respiratory distress syndrome) as this is the clinical finding most associated with poor outcomes in COVID-19. Mortality can vary when resources are stretched but ARDS is an outcome that we can’t yet prevent and its development should not be affected by the availability clinical resources. It is important that such endpoints have clinical significance for patients and healthcare providers.
What we have built is still in R&D mode (Research and Development), but we see this as a first step towards a fully functional software with AI capabilities. Most importantly we will need more data to fine-tune and validate this work. We will need more resources so that this can go live. The analytics process we have in place is very promising. It follows a full predictive analytics lifecycle that include data architecture, data preparation, modeling and validation. The most important next step, though, is to use data from hospitals in the US and elsewhere to retrain the algorithms in order for this to be able to be used in different settings.
Citation:
Xiangao Jiang, Megan Coffee, Anasse Bari, Junzhang Wang, Xinyue Jiang, Jianping Huang, Jichan Shi, Jianyi Dai, Jing Cai, Tianxiao Zhang, Zhengxing Wu, Guiqing He, Yitong Huang. Towards an Artificial Intelligence Framework for Data-Driven Prediction of Coronavirus Clinical Severity. CMC-Computers, Materials & Continua, 2020 DOI: 10.32604/cmc.2020.010691
[subscribe]
[last-modified]
The information on MedicalResearch.com is provided for educational purposes only, and is in no way intended to diagnose, cure, or treat any medical or other condition. Always seek the advice of your physician or other qualified health and ask your doctor any questions you may have regarding a medical condition. In addition to all other limitations and disclaimers in this agreement, service provider and its third party providers disclaim any liability or loss in connection with the content provided on this website.
Last Updated on May 12, 2020 by Marie Benz MD FAAD