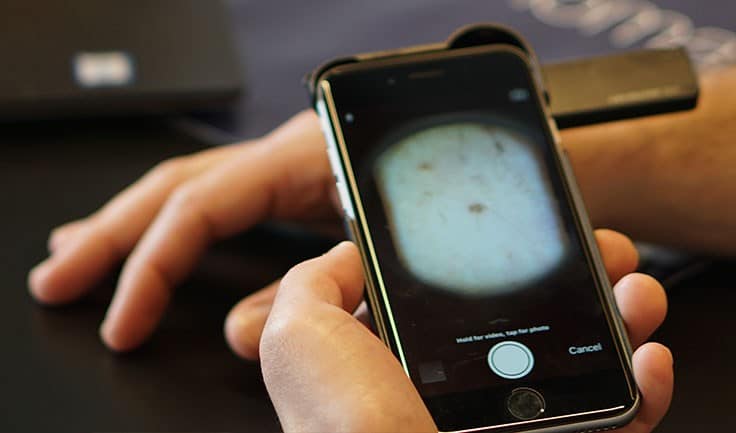
21 Oct DERM: AI Algorithm Helps Determine Likelihood of Melanoma from Dermoscopic Images
MedicalResearch.com Interview with:
Dr. Helen Marsden PhD
Skin Analytics Limited
London, United Kingdom
MedicalResearch.com: What is the background for this study?
Response: In this technology age, with the explosion of interest and applications using Artificial Intelligence, it is easy to accept the output of a technology-based test – such as a smartphone app designed to identify skin cancer – without thinking too much about it. In reality, technology is only as good as the way it has been developed, tested and validated. In particular, AI algorithms are prone to a lack of “generalisation” – i.e. their performance drops when presented with data it has not seen before. In the medical field, and particularly in areas where AI is being developed to direct a patient’s diagnosis or care, this is particularly problematic. Inappropriate diagnosis or advice to patients can lead to false reassurance, heightened concern and pressure on NHS services, or worse. It is concerning, therefore, that there are a large number of smartphone apps available that provide an assessment of skin lesions, including some that provide an estimate of the probability of malignancy, that have not been assessed for diagnostic accuracy.
Skin Analytics has developed an AI-based algorithm, named: Deep Ensemble for Recognition of Malignancy (DERM), for use as a decision support tool for healthcare providers. DERM determines the likelihood of skin cancer from dermoscopic images of skin lesions. It was developed using deep learning techniques that identify and assess features of these lesions which are associated with melanoma, using over 7,000 archived dermoscopic images. Using these images, it was shown to identify melanoma with similar accuracy to specialist physicians. However, to prove the algorithm could be used in a real life clinical setting, Skin Analytics set out to conduct a clinical validation study.
MedicalResearch.com: What are the main findings?
Response: The study was run in dermatology and plastics clinics across 7 UK Hospital Trusts. 514 patients who had at least one pigmented skin lesion that a specialist referred for biopsy, were recruited. Photographs of these lesions, plus two known benign lesions and a patch of clear skin, were taken using two smartphone cameras (an iPhone 6S and a Samsung Galaxy S6), and a DSLR camera, all with dermoscopic lens attachments. The histopathology-confirmed diagnosis was used as the “true diagnosis” against which DERM was compared. The study also included an assessment of the diagnostic accuracy of skin cancer specialists against the histologically-confirmed diagnosis, and the collection of a separate image set that could be used to further train the algorithm.
DERM produces a numerical output from zero to one, which reflects its ‘confidence’ that the lesion is melanoma. A decision threshold defines the point above which a lesion is classed as melanoma. The decision threshold can be set to a specific sensitivity or specificity level, to allow for comparison against other diagnostic methodologies, or to balance the false positive (over referral) and false negative (missed diagnosis) rates appropriate for the setting in which DERM is being used.
The number of unnecessary referrals for suspected skin cancer is a major problem for the NHS, with many doctors throughout the patient pathway taking a “better safe than sorry” approach to their patients’ health. Therefore, for a technology to be useful to the NHS, it needs to be able to reduce the number of unnecessary referrals, without missing any malignant lesions.
When the decision threshold was set on the best performing version of the algorithm, using images from the iPhone camera, to match that of skin cancer specialists, DERM was able to identify all the malignant cases but identified fewer false positive cases than the specialists, in those lesions referred for biopsy. However, it did also falsely identify melanoma in lesions that were clearly benign.
MedicalResearch.com: What should readers take away from your report?
Response: Robust studies designed to critically assess the diagnostic accuracy of an AI technology are few and far between, but any new technology being launched into clinical practice must be fully tested. AI, in particular, function as a “black box” and without fully evaluating a product, healthcare professionals (and consumers, where technology is made available to patients directly) are unlikely to be aware of the potential for inaccurate results from the technology.
We believe this is the first fully powered study to formally test the diagnostic accuracy of a melanoma-detection algorithm. The study showed the algorithm can detect melanoma with a similar level of accuracy to specialists. The development of low-cost screening methods, such as AI-based services, could transform patient diagnosis pathways, enabling greater efficiencies throughout the healthcare service.
MedicalResearch.com: What recommendations do you have for future research as a result of this work?
Response: The results of this study provide the first pillar of evidence for the use of DERM in clinical practice. Additional studies on the diagnostic accuracy of the algorithm to detect other conditions (such as Non-Melanoma Skin Cancers, and benign conditions often mistaken for more concerning), and an initial assessment on the impact DERM could have in practice, and a primary-care based study are about to be launched.
We believe the healthcare community should expect any technology, wherever deployed in the health service, to be able to produce high quality evidence to support the use of that technology in the setting it is deployed.
MedicalResearch.com: Is there anything else you would like to add?
Response: This research would not have been possible without the patients who agreed to consent to the study, and the hospital staff who collected the images. The study was funded by Skin Analytics, who developed DERM.
Citation:
Phillips M, Marsden H, Jaffe W, et al. Assessment of Accuracy of an Artificial Intelligence Algorithm to Detect Melanoma in Images of Skin Lesions. JAMA Netw Open. Published online October 16, 20192(10):e1913436. doi:10.1001/jamanetworkopen.2019.13436
https://jamanetwork.com/journals/jamanetworkopen/fullarticle/2752995
[wysija_form id=”3″]
[last-modified]
The information on MedicalResearch.com is provided for educational purposes only, and is in no way intended to diagnose, cure, or treat any medical or other condition. Always seek the advice of your physician or other qualified health and ask your doctor any questions you may have regarding a medical condition. In addition to all other limitations and disclaimers in this agreement, service provider and its third party providers disclaim any liability or loss in connection with the content provided on this website.
Last Updated on October 21, 2019 by Marie Benz MD FAAD