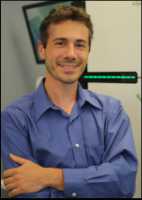
17 Sep Artificial Intelligence Can Reliably Diagnosis Specific Types of Lung Cancer
MedicalResearch.com Interview with:
Aristotelis Tsirigos, Ph.D.
Associate Professor of Pathology
Director, Applied Bioinformatics Laboratories
New York University School of Medicine
MedicalResearch.com: What is the background for this study? What are the main findings?
Response: Pathologists routinely examine slides made from tumor samples to diagnose cancer types. We studied whether an AI algorithm can achieve the same task with high accuracy. Indeed, we show that such an algorithm can achieve an accuracy of ~97%, slightly better than individual pathologists.
In addition, we demonstrated that AI can be used to predict genes that are mutated in these tumors, a task that pathologists cannot do. Although the accuracy for some genes is as high as 86%, there is still room for improvement. This will come from collecting more training data and also from improvement in the annotations of the slides by expert pathologists.
MedicalResearch.com: What should readers take away from your report?
Response: AI can reliably diagnose specific types of lung cancer based on slides. This does not mean that doctor will be replaced any time soon, but when used in clinical practice it can – at the very least – help pathologists reduce human error.
MedicalResearch.com: What recommendations do you have for future research as a result of this work?
Response: In this study, we showed that AI can be used to make a diagnosis. Naturally, the next question is whether it can also be used to determine clinical outcomes, such as response to therapy and patient survival. Our team is now also focusing on this very important aspect.
No disclosures.
Citation:
Nicolas Coudray, Paolo Santiago Ocampo, Theodore Sakellaropoulos, Navneet Narula, Matija Snuderl, David Fenyö, Andre L. Moreira, Narges Razavian, Aristotelis Tsirigos. Classification and mutation prediction from non–small cell lung cancer histopathology images using deep learning. Nature Medicine, 2018; DOI: 1038/s41591-018-0177-5
[wysija_form id=”3″]
[last-modified]
The information on MedicalResearch.com is provided for educational purposes only, and is in no way intended to diagnose, cure, or treat any medical or other condition. Always seek the advice of your physician or other qualified health and ask your doctor any questions you may have regarding a medical condition. In addition to all other limitations and disclaimers in this agreement, service provider and its third party providers disclaim any liability or loss in connection with the content provided on this website.
Last Updated on September 17, 2018 by Marie Benz MD FAAD