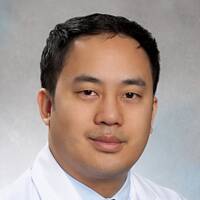
07 Sep Lung Cancer: Human-AI Collaboration Can Accelerate Time to Treatment
MedicalResearch.com Interview with:
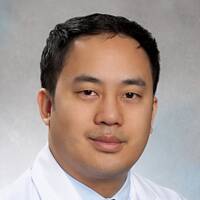
Dr. Mak
Raymond H. Mak, MD
Radiation Oncology Disease Center Leader for Thoracic Oncology
Director of Patient Safety and QualityDirector of Clinical Innovation
Associate Professor, Harvard Medical School
Cancer – Radiation Oncology, Radiation Oncology
Department of Radiation Oncology
Brigham and Women’s Hospital
MedicalResearch.com: What is the background for this study? What is the algorithm detecting?
Response: Lung cancer, the most common cancer worldwide is highly lethal, but can be treated and cured in some cases with radiation therapy. Nearly half of lung cancer patients will eventually require some form of radiation therapy, but the planning for a course of radiation therapy currently entails manual, time-consuming, and resource-intensive work by highly trained physicians to segment (target) the cancerous tumors in the lungs and adjacent lymph nodes on three-dimensional images (CT scans). Prior studies have shown substantial variation in how expert clinicians delineate these targets, which can negatively impact outcomes and there is a projected shortage of skilled medical staff to perform these tasks worldwide as cancer rates increase.
To address this critical gap, our team developed deep learning algorithms that can automatically target lung cancer in the lungs and adjacent lymph nodes from CT scans that are used for radiation therapy planning, and can be deployed in seconds.
We trained these artificial intelligence (AI) algorithms using expert-segmented targets from over 700 cases and validated the performance in over 1300 patients in external datasets (including publicly available data from a national trial), benchmarked its performance against expert clinicians, and then further validated the clinical usefulness of the algorithm in human-AI collaboration experiments that measured accuracy, task speed, and end-user satisfaction.
MedicalResearch.com: What are the main findings?
Response: We demonstrated that our AI algorithm could automatically segment/target lung cancer in the chest (both primary lung tumor and lymph nodes) with a high level of accuracy. As a benchmark, we volumetrically compared the AI-generated segmentations against the gold standard expert-delineated targets and found that the overlap was within the variation we observe between human clinicians.
Most importantly, we demonstrated that these volumetric overlap metrics (e.g. Dice Coefficient) that are commonly used for in silico validation of auto-segmentation algorithms did not correlate well with clinical utility (e.g. task speed improvement, end-user satisfaction) by conducting extensive end-user testing and survey work.
In these human-AI collaboration experiments, we asked clinicians to either manually segment/target lung cancer cases de novo, or to edit a segmentation we provided them in a clinical environment. We added a wrinkle to the study by providing the clinicians with either an AI-generated segmentation or a segmentation generated by another clinician, but in a blinded fashion. In this three-way comparison, we found that AI-collaboration led to a 65% reduction in segmentation time (median 15.5 minutes to 5.4 minutes) and 32% reduction in inter-clinician variation, but interestingly, the clinicians did not experience a significant time savings when editing another human’s segmentation.
MedicalResearch.com: What should readers take away from your report?
Response: One of the biggest translation gaps in AI applications to medicine is the failure to study how to use AI to improve human clinicians, and vice versa. In this study, we took advantage of clinician expertise and intuition with early input into the development and training of the AI algorithm. Essentially, we first had human teach AI by studying how early iterations of the algorithms were failing and then augmenting our training data to improve performance.
We then demonstrated that the final AI algorithms can actually improve human performance in a human-AI partnership that can result in a direct benefit to patients through greater consistency in segmenting tumors and accelerating times to treatment. Our surveys of the clinicians who partnered with the AI demonstrate that they also experienced substantial benefits in reduced task time, high satisfaction and reduced perception of task difficulty, which is an interesting additional benefit that we had not thought about initially… that AI could reduce cognitive load and possibly reduce physician burnout. Hopefully, as we deploy these algorithms into the clinic, we will also see additional benefits for clinicians with reduced time doing mundane computer work, and more time in quality interactions with patients.
MedicalResearch.com: What recommendations do you have for future research as a results of this study?
Response: For readers that are evaluating new AI technologies for clinical implementation, we hope that this manuscript presents a framework for thoughtful AI development that incorporates clinician input and includes a rigorous testing and validation framework including performance benchmarking, identifying of key failures modes and determine whether an AI algorithm performs as intended in the hands of clinicians, before introduction of the algorithm in the clinic. We believe that an evaluation strategy for AI models that emphasizes the importance of human-AI collaboration is especially necessary because in silico (computer-modeled) validation can give different results than clinical evaluations. As an extension of this work, we are designing and conducting prospective, randomized trials of similar AI auto-segmentation algorithms in the clinic to provide the highest level of evidence.
MedicalResearch.com: Is there anything else you would like to add?
Response: Keep an eye out for our upcoming work where we will convert this AI development, testing and validation framework into an “AI label”, which we hope will provide radiation oncology researchers and clinicians with a way to quickly reference and understand the core components, performance features, and “warnings” of a given algorithm (like a FDA drug label).
Disclosures: Disclosures: Dr. Mak received research funding from ViewRay, Inc. and honoraria from NewRT and ViewRay, Inc., is on the Advisory Board of AstraZeneca and ViewRay, is a scientific advisor and shareholder of Health-AI, Inc., and received travel stipends from NewRT and ViewRay. A complete list of other author disclosures is available online.Funding: This study was funded by the National Institutes of Health (U24CA194354, U01CA190234, and U01CA209414).
Citation:
Hosny A et al. “Clinical Validation of Deep Learning Algorithms for Lung Cancer Radiotherapy Targeting” Lancet Digital Health DOI: 10.1016/ S2589-7500(22)00129-7
https://linkinghub.elsevier.com/retrieve/pii/S2589750022001297
The information on MedicalResearch.com is provided for educational purposes only, and is in no way intended to diagnose, cure, or treat any medical or other condition. Always seek the advice of your physician or other qualified health and ask your doctor any questions you may have regarding a medical condition. In addition to all other limitations and disclaimers in this agreement, service provider and its third party providers disclaim any liability or loss in connection with the content provided on this website.
Last Updated on September 7, 2022 by Marie Benz MD FAAD