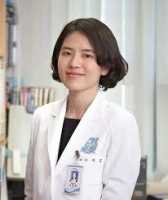
03 Apr AI Improved Diagnosis of Skin Disorders, especially Distinguishing Benign from Malignant Tumors
MedicalResearch.com Interview with:
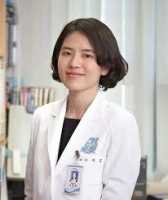
Dr. Jung Im Na
Jung-Im Na, MD PhD
Associate Professor, Department of Dermatology
Seoul National University Bundang Hospital
Korea
MedicalResearch.com: What is the background for this study? Would you briefly explain what is meant by a convolutional neural network?
Response: When a very young child looks at a picture, she can easily identify cats and dogs, however, even the most advanced computers had struggled at this task until recently.
Computers began to “see” with the recent advancement of Deep Learning techniques. Deep Learning is a machine learning technique that teaches computers to learn from raw data. Most deep learning methods use artificial neural network architectures, imitating human brain, and convolutional neural networks (CNN) is a particular type of deep learning architecture, imitating the visual cortex. CNN is especially powerful for recognizing images. CNN exploit the information contained in image datasets to automatically learn features and patterns.
MedicalResearch.com: What are the main findings? How might dermatologists employ this system in real practice?
Response: Several previous studies have demonstrated the potential of deep learning-based AI system to be used for diagnostic dermatology. Most of these studies, however, have focused on highlighting the performance of AI with a focus on its equivalent or superior diagnostic ability compared to human experts. Moreover, these comparisons have been limited to specific binary tasks, such as discriminating malignant versus benign lesion. However, an algorithm for specific purposes has inevitable limitations. For example, an algorithm trained only to differentiate between melanoma and nevi may misdiagnose inflamed acne as melanoma.
For these systems to be practically useful, they’re required to identify various skin disorders and their performance need to be tested in an environment similar to real practice, which demands not only classifying malignant versus benign lesion, but also distinguishing skin cancer from numerous other skin disorders including inflammatory and infectious conditions.
We collected 220,000 images of Asians and Caucasians with 174 skin diseases and trained neural networks to interpret those images. The algorithm we developed was able to accurately predict malignancy, suggest primary treatment options, and render multi-class classification among 134 skin disorders, which is the largest number of disease classes among studies applying deep learning to medical research.
More importantly, the algorithm improved the performance of medical professionals in skin disease diagnosis. We demonstrated that, in the malignancy and treatment option prediction as well as multi-disease classification task, both dermatologists and dermatology residents benefitted with an assistance from our algorithm. The algorithm suggests three most possible diagnosis with 69% of accuracy, predict malignancy and suggests treatment options. Dermatologists can refer to the algorithm’s suggestion, which will greatly ease the diagnosing process. The algorithm will be even more helpful for non-dermatologists, who have less knowledge of skin diseases.
Among various skin diseases, the algorithm is especially useful for diagnosing benign and malignant tumors. This is because, unlike other skin diseases, tumors can be pictured easily with appropriate composition. Non-dermatologists and even general public can take picture of a tumor and check if it’s malignant. In our study, the sensitivity of the diagnosis of malignancy by the 23 members of the general public was only 47.6 %, which means half of the malignancies would have been missed by the general public without referral to specialists. With the help of the algorithm, the sensitivity markedly increased to 87.5%. We expect that the use of our algorithm with a smartphone may be able to encourage the public to visit specialists for cancerous lesions, such as melanoma, that might have been otherwise neglected.
MedicalResearch.com: What should readers take away from your report?
Response: Although several previous studies demonstrated algorithms the dermatologist-level diagnosing performance, the reproducibility is still a challenge. Since the neural network has uncertainty toward untrained data, it can produce unexpected output. Considering the characteristics of the neural network, it needs to be validated by prospective randomized study in real practice. It is necessary to confirm whether the algorithm’s performance is reasonable in actual practice settings as well as in study settings.
MedicalResearch.com: What recommendations do you have for future research as a result of this work?
Response: AIs depicted in media appear far smarter than humans. In fact, they are not. AIs outperform humans only in limited conditions. To get the best use of them, we need to understand tasks appropriate for AIs. AI research is currently in its infancy, and many researchers are working to expand its limited conditions. AI research requires domain knowledge and computing skills to proceed. For example, although our team has computing skills, we’ll not be able to develop AI for X-ray reading because of lack of domain knowledge for radiology. For the most efficient AI research, it is desirable for researcher to have both domain knowledge and computer skills. Therefore basic researchers such as biologists and doctors should be interested in computing skills.
This study was performed without the help of company. Dr. Han who trained the algorithm is a dermatologists. He also developed the website, in which we released a demo version of our algorithms. The website (http://modelderm.com) is freely accessible by PCs and smartphones. We hope that our effort can facilitate scientific communications among researchers.
Citation:
Seung Seog Han, … Jung-Im Na
JID: In Press, Corrected Proof, Available online 31 March 2020
[subscribe]
[last-modified]
The information on MedicalResearch.com is provided for educational purposes only, and is in no way intended to diagnose, cure, or treat any medical or other condition. Always seek the advice of your physician or other qualified health and ask your doctor any questions you may have regarding a medical condition. In addition to all other limitations and disclaimers in this agreement, service provider and its third party providers disclaim any liability or loss in connection with the content provided on this website.
Last Updated on April 3, 2020 by Marie Benz MD FAAD